Bullseye Faces Viewer Criticism
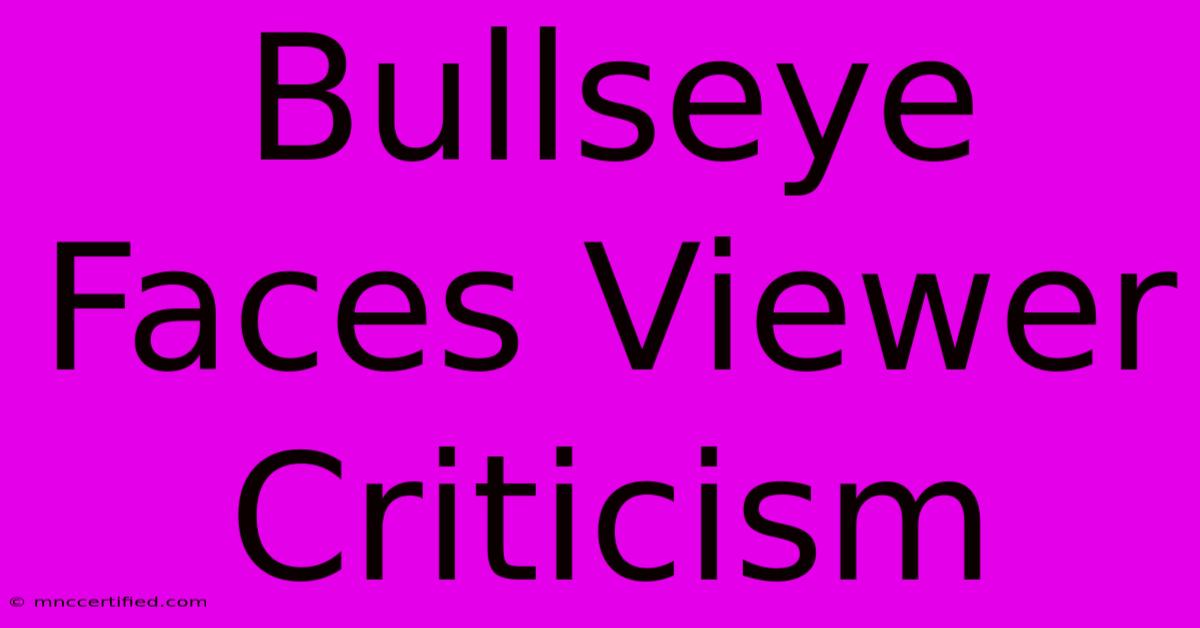
Table of Contents
Bullseye Faces Viewer Criticism: A Deep Dive into the Controversy
Bullseye, the popular facial recognition software, has recently faced a wave of criticism from users and privacy advocates alike. This article delves into the specifics of this controversy, examining the various concerns raised and exploring the potential implications for the future of facial recognition technology.
Accuracy and Bias Concerns: The Core of the Criticism
One of the most significant criticisms leveled against Bullseye is its accuracy. While the software boasts impressive capabilities, users have reported instances of misidentification, leading to incorrect tagging and potentially serious consequences. This inaccuracy is exacerbated by concerns about bias within the algorithm. Studies have suggested that facial recognition systems, including Bullseye, may be less accurate in identifying individuals with darker skin tones or certain facial features. This inherent bias raises significant ethical concerns, particularly in applications involving law enforcement and security. The lack of transparency regarding the datasets used to train the algorithm further fuels these anxieties. Users are demanding more information about how the system functions and the steps being taken to address these biases.
The Impact of Misidentification: Real-World Consequences
The consequences of inaccurate facial recognition can be severe. Misidentification in security applications could lead to wrongful arrests or denials of access. In social media contexts, it could result in the wrong individuals being tagged in photos or videos, potentially leading to reputational damage or even harassment. These real-world consequences highlight the urgent need for improved accuracy and a robust system for addressing errors.
Privacy Violations: A Growing Concern
Beyond accuracy concerns, the privacy implications of Bullseye are also a major source of criticism. The collection and storage of vast amounts of facial data raise significant questions about data security and potential misuse. Users worry about the potential for this data to be leaked, hacked, or used for purposes beyond what was initially intended. The lack of clear consent mechanisms and robust data protection measures further fuels these concerns.
The Need for Transparency and User Control
Many critics argue that Bullseye lacks sufficient transparency regarding its data practices. Users need clear and accessible information about how their data is being collected, stored, and used. Furthermore, they demand greater control over their own data, including the ability to opt out of facial recognition entirely or to delete their data from the system.
Addressing the Criticism: Moving Forward
The criticism directed at Bullseye is not merely a fleeting trend; it represents a growing awareness of the ethical and practical challenges posed by facial recognition technology. To regain user trust and ensure responsible development, Bullseye's developers need to address the following:
- Improve Accuracy and Reduce Bias: Invest in research and development to improve the algorithm's accuracy and eliminate bias. This includes using more diverse and representative datasets for training and implementing rigorous testing procedures.
- Enhance Transparency and Data Security: Provide clear and concise information about data collection, storage, and usage practices. Implement robust security measures to protect user data from unauthorized access and breaches.
- Implement Strong User Controls: Give users greater control over their data, allowing them to opt out of facial recognition or delete their data at any time.
- Engage in Open Dialogue: Actively engage with critics and stakeholders to address concerns and build trust.
The future of facial recognition technologies like Bullseye hinges on addressing these criticisms effectively. Ignoring these concerns will only serve to exacerbate the controversy and potentially undermine the technology's long-term viability. The focus must shift towards developing responsible, ethical, and user-centric systems that prioritize accuracy, privacy, and transparency.
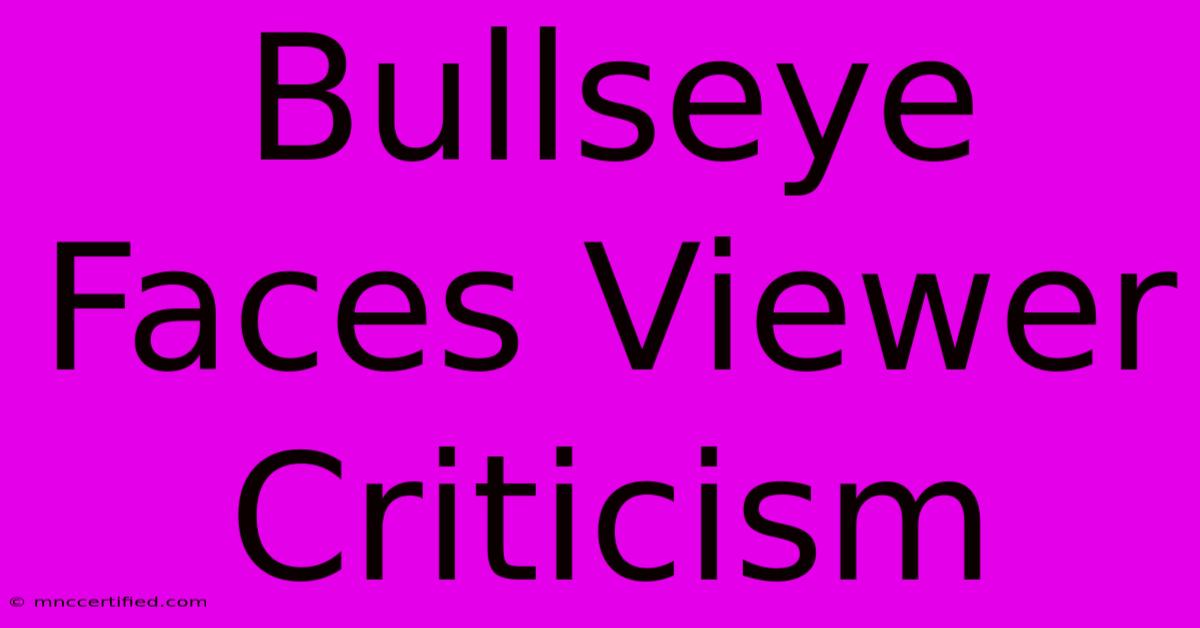
Thank you for visiting our website wich cover about Bullseye Faces Viewer Criticism. We hope the information provided has been useful to you. Feel free to contact us if you have any questions or need further assistance. See you next time and dont miss to bookmark.
Featured Posts
-
Belfast Airport Runway Remains Closed After Aer Lingus
Dec 23, 2024
-
Bournemouth Vs Man Utd Predicted Team
Dec 23, 2024
-
20 Classic Mariah Carey Songs
Dec 23, 2024
-
Lions Injury Update Detroit Vs Chicago
Dec 23, 2024
-
Trumps Panama Canal Plan
Dec 23, 2024